A Learned Radiance-Field Representation for Complex Luminaires
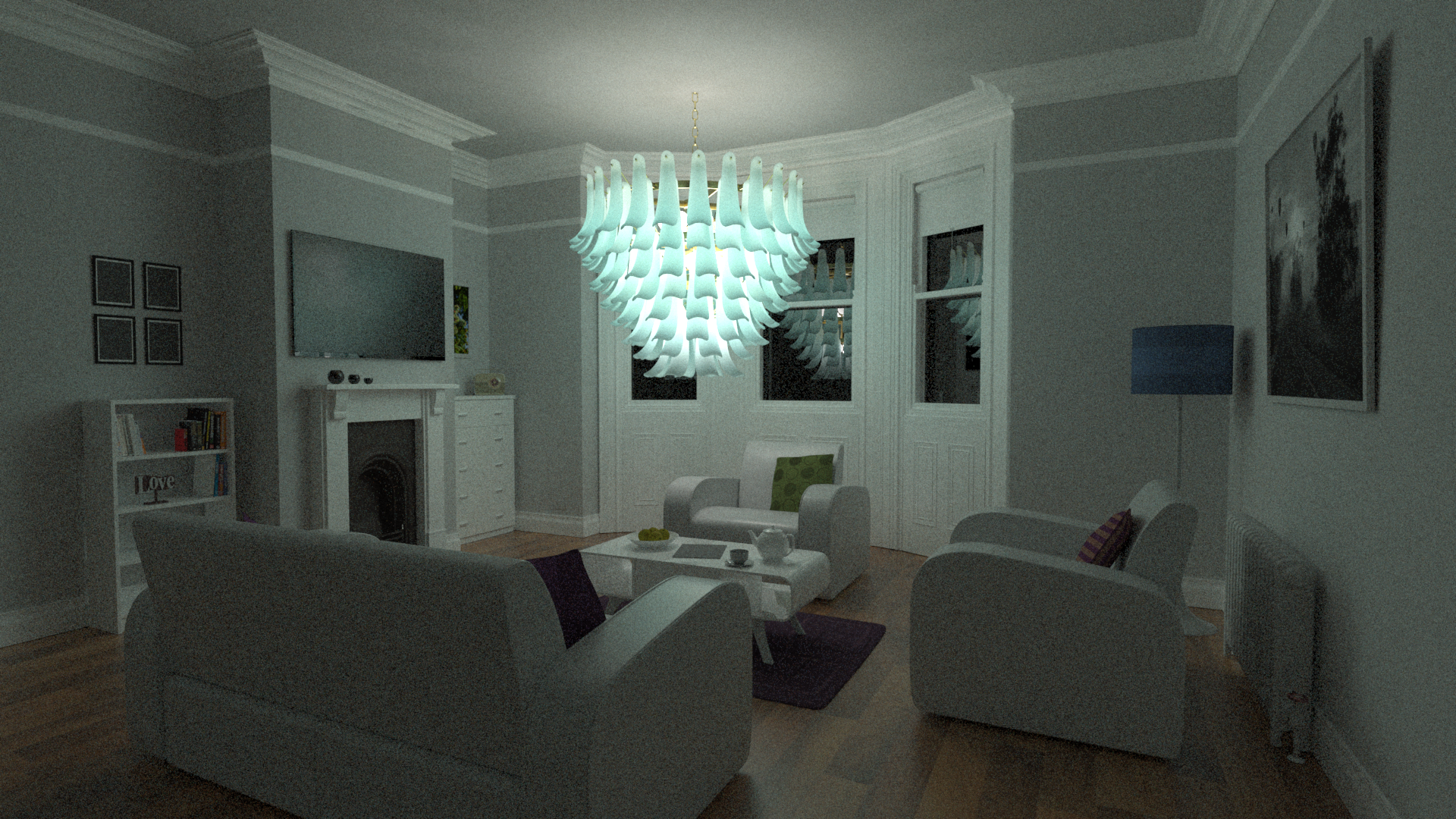
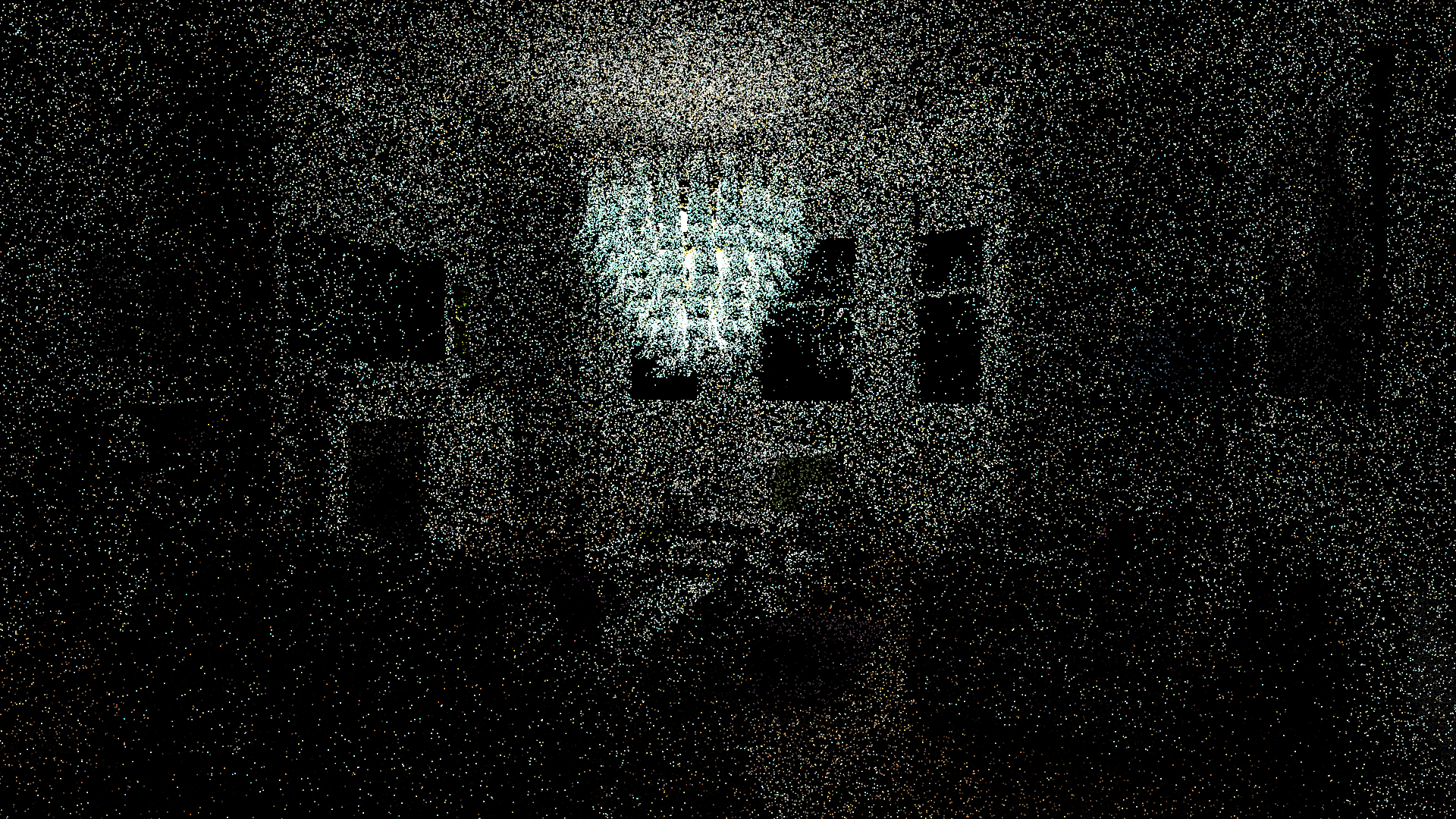
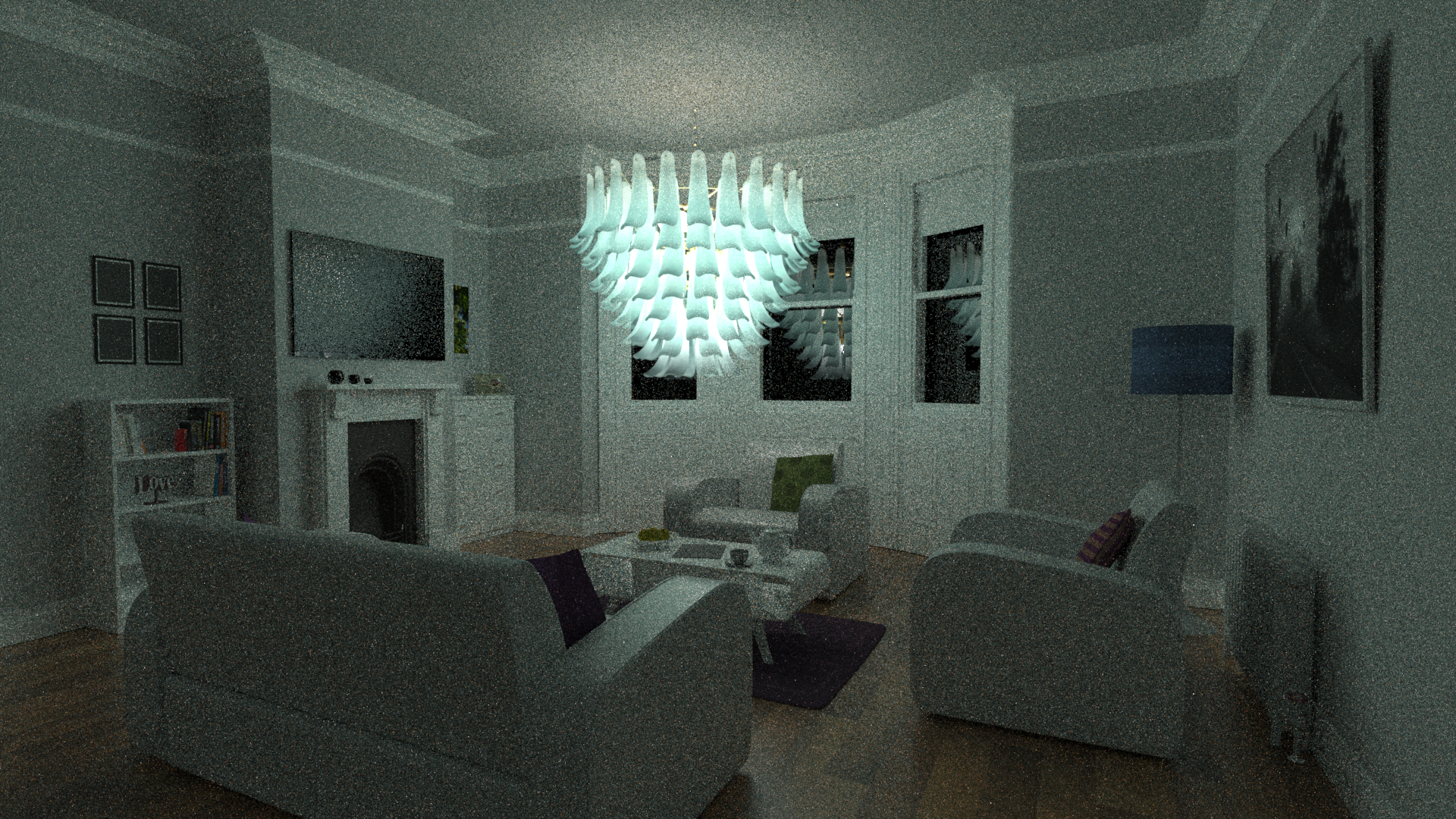
We propose an efficient method for rendering complex luminaires using a high quality octree-based representation of the luminaire emission. Complex luminaires are a particularly challenging problem in rendering, due to their caustic light paths inside the luminaire. We reduce the geometric complexity of luminaires by using a simple proxy geometry, and encode the visually-complex emitted light field by using a neural radiance field. We tackle the multiple challenges of using NeRFs for representing luminaires, including their high dynamic range, high-frequency content and null-emission areas, by proposing a specialized loss function. For rendering, we distill our luminaires' NeRF into a plenoctree, which we can be easily integrated into traditional rendering systems. Our approach allows for speed-ups of up to 2 orders of magnitude in scenes containing complex luminaires introducing minimal error.
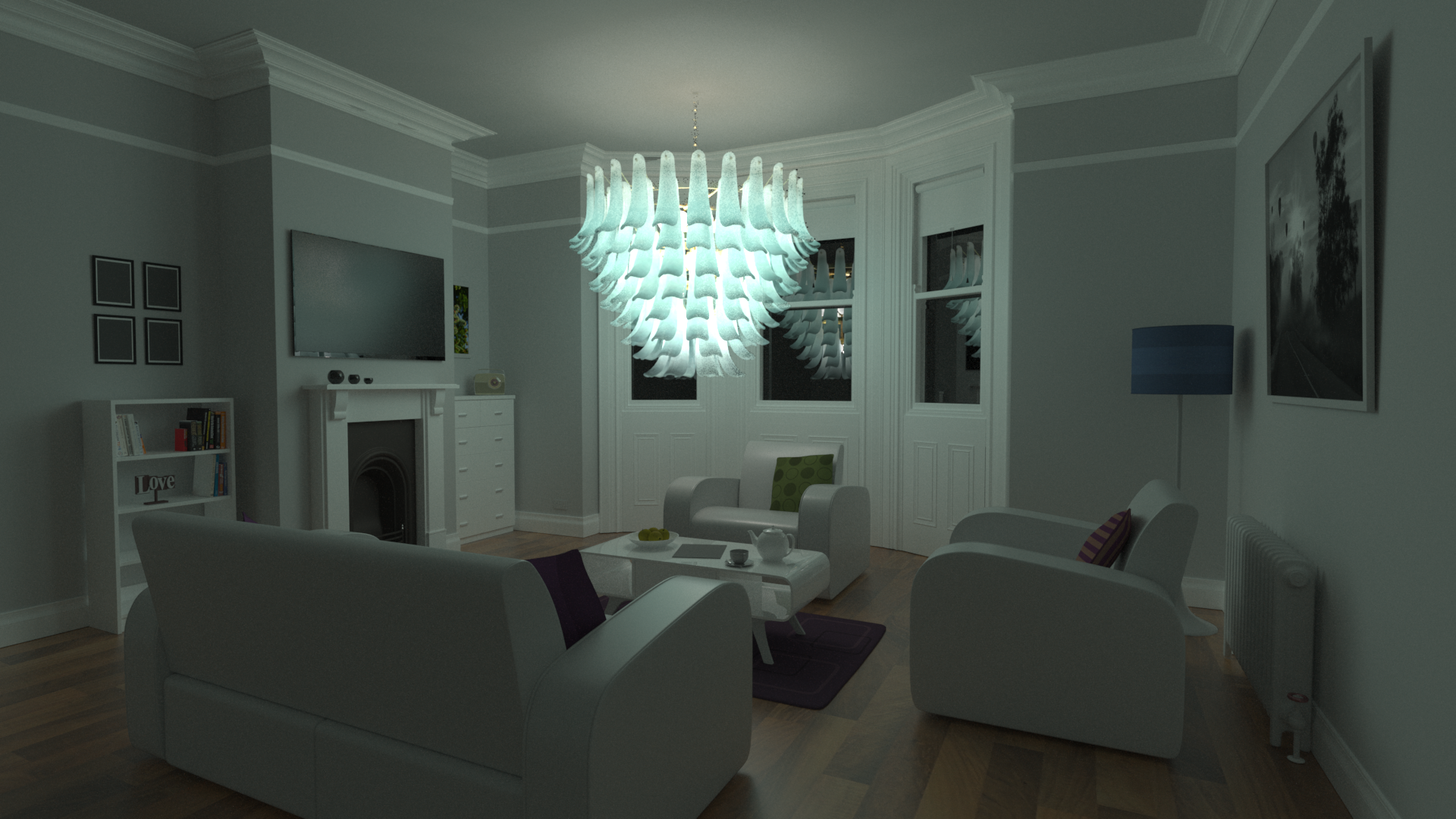
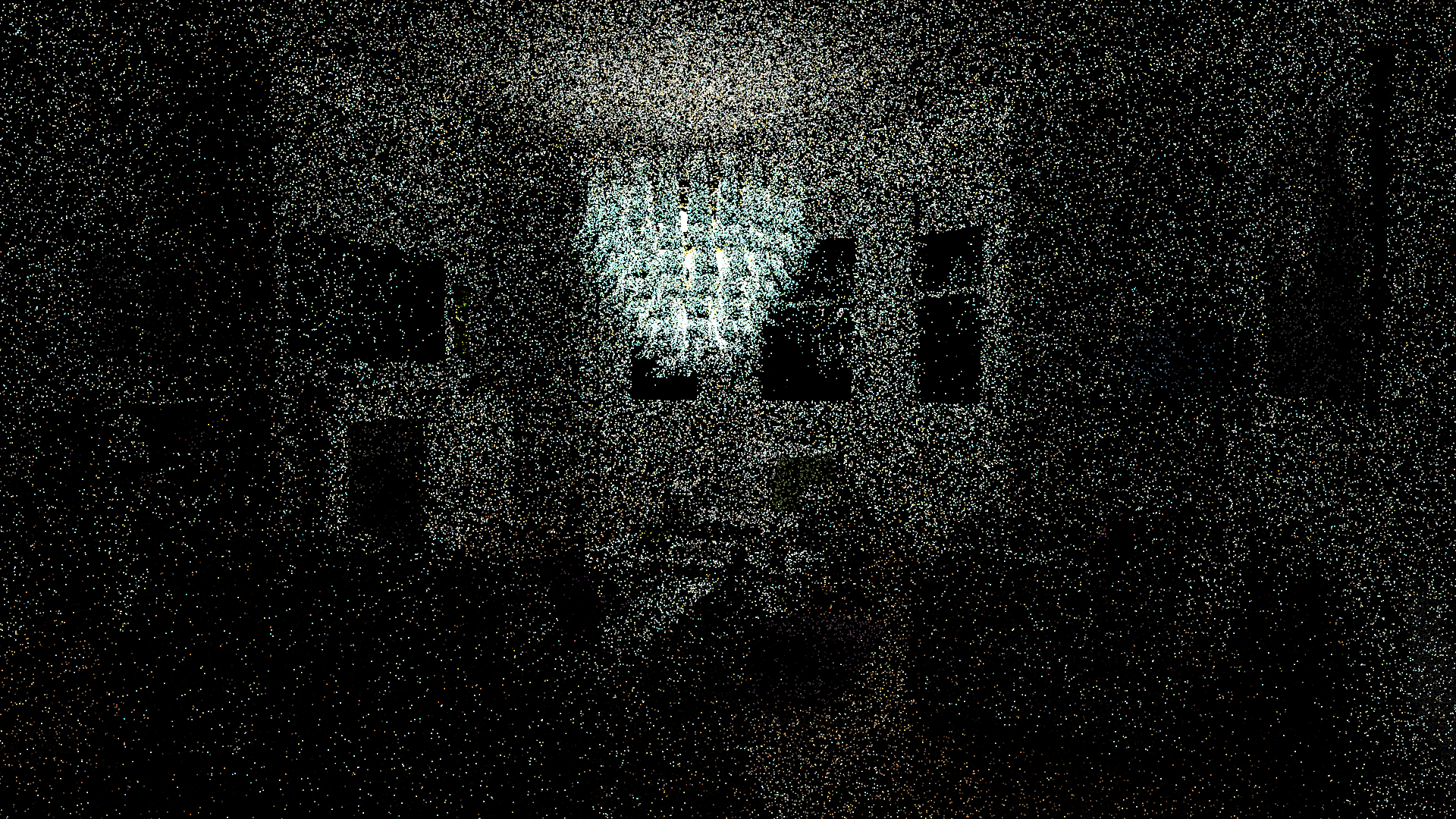
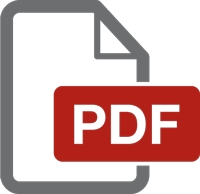
Jorge Condor, Adrián Jarabo, A Learned Radiance-field Representation for Complex Luminaires, EGSR Conference Proceedings (2022)
@inproceedings {Condor2022,
booktitle = {Eurographics Symposium on Rendering},
editor = {Ghosh, Abhijeet and Wei, Li-Yi},
title = {{A Learned Radiance-Field Representation for Complex Luminaires}},
author = {Condor, Jorge and Jarabo, Adrián},
year = {2022},
publisher = {The Eurographics Association},
ISSN = {1727-3463},
ISBN = {978-3-03868-187-8},
DOI = {10.2312/sr.20221155}
}